#1 Ranking: Read how InetSoft was rated #1 for user adoption in G2's user survey-based index |
|
Read More |
In disciplines including urban planning, environmental monitoring, and geology, spatial data is often employed. To better understand how buildings, roads, and other infrastructure interact with one another and the environment, urban planners, for instance, utilize spatial data to map out the positions of these features. Spatial data is used by environmental scientists to monitor the movement of contaminants and analyze the effects of natural catastrophes on the environment.
Time-Series Information
Data that is gathered over time is referred to as time-series data. It may consist of measurements of a single variable taken at several times or of many variables that vary over time. Stock prices, weather information, and sensor readings are a few examples of time-series data.
In disciplines including engineering, economics, and finance, time-series data is often employed. Economists, for instance, examine historical patterns in inflation or unemployment rates using time-series data. Engineers utilize time-series data to track the functioning of equipment and forecast when maintenance or repairs will be required.
Image Information
Data that represents visual information, such as pictures, movies, and digital images, is referred to as image data. Together with their location and size, it may also provide details like the color, brightness, and texture of the items. In disciplines including computer vision, medical imaging, and remote sensing, image data is often employed.
Computer vision researchers utilize visual data to create algorithms that can identify objects and situations in pictures and movies. Image data is used by medical imaging experts to diagnose illnesses and injuries as well as to direct surgical operations. Researchers that study the Earth's surface and atmosphere using remote sensing also utilize picture data to track changes in vegetation and land use.
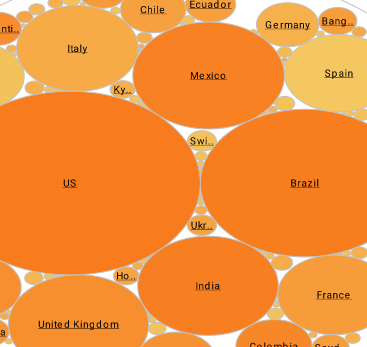 |
“Flexible product with great training and support. The product has been very useful for quickly creating dashboards and data views. Support and training has always been available to us and quick to respond.
- George R, Information Technology Specialist at Sonepar USA
|
Text Information
Text data is information that is expressed via spoken or written words. It may include details on the terms used, their definitions, and how they relate to one another. Natural language processing, sentiment analysis, and social network analysis are just a few of the disciplines that often employ text data.
Researchers in natural language processing employ text data to create algorithms that can comprehend and produce human language. Researchers that study sentiment employ text data to examine the emotional undertone of spoken or written language, such as that used in social media postings or product evaluations. Text data is used by social network analysis researchers to examine how individuals connect and communicate online.
Chart Data
Data that depicts networks or connections between items is referred to as graph data. It may include details on the network's nodes, or vertices, as well as its edges, or connections. Graph data is often utilized in disciplines including social network research, biology, and transportation planning.
Graph data is used by social network researchers to examine the connections between users of social networks like Facebook and Twitter. Graph data is used by bioinformaticians to investigate the interactions between genes and proteins in biological systems. The flow of cars and people via road networks and public transit systems is modeled by transportation planners using graph data.
Multidimensional Data Applications
Predictive Modeling
Statistical and machine learning approaches are used in predictive modeling to create predictions based on data. Since it enables researchers to take into account several variables that can have an impact on the result of interest, multidimensional data is often employed in predictive modeling.
For instance, a business may create a prediction model for future sales using time-series data on customer purchases, website traffic, and social media activity. The business may find patterns and associations that can be helpful in projecting future client behavior by studying these many data components.
Data Visualization
Data visualization is the process of presenting complicated data in a simple and understandable manner using graphs, charts, and other visual representations. Since it enables analysts to display several elements and connections in a single picture or graph, multidimensional data is often utilized in data visualization.
A scientist researching climate change, for instance, would utilize geographical data to create a map that depicts the spread of carbon emissions over the globe. The scientist may see patterns and trends that might not be obvious from raw data by displaying this multidimensional data.
Clustering and Classification
Clustering and classification are terms used to describe the use of machine learning algorithms to classify or predict the class or category of a new data point. Since it enables researchers to take into account several characteristics that may be important when grouping or classifying data, multidimensional data is often utilized in clustering and classification.
For instance, a store may create a clustering algorithm that puts related goods together based on the preferences and traits of the customers using text data from customer reviews and product descriptions. The merchant may create a more precise clustering algorithm by examining many aspects of data, such as the language used in reviews, the product price, and consumer demographics.
Finding Anomalies
Anomaly detection is the process of identifying data items that substantially depart from the norm using statistical and machine learning approaches. Since it enables analysts to take into account several variables that may be important in recognizing anomalous data points, multidimensional data is often employed in anomaly identification.
For instance, a bank may analyze time-series information on client transactions to spot fraud. The bank may create a more precise algorithm for identifying fraud by examining many aspects of data, such as the location of the transaction, the time of day, and the amount of money involved.