Maybe there are some change requests, and we are supporting this as an experimental business intelligence app
in a limited way or in a piloted way. Now, out of those, maybe some of those solutions become quite valuable,
and the way we have integrated this data and analyzed it. It’s so useful that we want to publish this as
system of record content to a wider audience.
And with each tier, there is more and more rigor and sanctioning of this information as yeah, this is valid
consensus information. We just kind of go from conjecture at the bottom to consensus at the top and eventually
we get to content that is published and ready to be disseminated.
And I think this is a real key, is real training and impress upon your users and your stakeholders the rule
of an engagement that goes along with using information at each level or each tier. You might want to train
users to be able to qualify them to build prototypes that can be subsequently promoted to further levels of
consensus information.
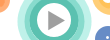 |
View a 2-minute demonstration of InetSoft's easy, agile, and robust BI software. |
So that’s the second key issue on data discovery and the technology. Clearly, the semantic layer based
BI platforms that a lot of organizations have been using have been challenged by data discovery. And I think
we talked about in detail why they are being challenged. It’s that rapid prototyping capability, it's
that unfettered drilling capability, and it’s that really compelling modern looking interface
that’s very intuitive to use.
I think it's combination of all three of those, but particularly the data mashup really which gives that
rapid prototyping and that agility that folks want that’s just disrupting the classic semantic layer and
data warehouse architecture. So as we introduce these tools, I think we want to introduce them in a way that
doesn’t throw out the baby with the bath water and keeps some form of governance and consistency.
Because as much as companies may need agility and responsiveness and to see information quickly, they also
need to be able to say with authority and confidence that these are the dimensions and measures that describe
my business, and this is what we are running on. And those last couple of slides, where we talked about
promoting content and having tiered certification on prototype, pilot and production, I think it’s a
good way you can have your cake and eat it too where we can deliver that agility and still remain governed and
consistent information.
Okay, let’s move on the last key issue. We are talking about the organizational model. And here is a
business analytics framework. There is a people process and technology or a platform pillar and people look at
this and kind of say okay yeah, there is information management layer, and oh there’s their analytical
capabilities layer and that’s on Microstrategy or Cognos or something.
I think this picture is a much more accurate picture where yeah, there is a centralized team of very
confident people who know how to integrate and model data, and they’ve got a centralized data warehouse
architecture like that first slide I showed you, but there is a whole lot of information being integrated,
analyzed, and applied to the decisions in these companies that have got nothing to do with our centralized
team.
Whether it's in the sales, service, marketing, HR, finance, and all the horizontal functions, whether it's in
different lines of business units or different geographies, there is a lot of analytics going on that are
autonomous and independent, and I think this is the picture that we have today. And as sort of a homework for
you, I think as you have listened to me for an hour today, is to write what this document looks like for you
guys.
I don’t know if it's two or three decentralized teams, six or seven decentralized teams or whether you
have dozens and dozens of decentralized teams, but I would like you to get a blank piece of paper and draw
this picture. You know, hey, here is our centralized team blending these different skill sets together to do
BI centralizing, but where are all our de-centralized teams? How many do I have? What type of skill sets do I
have in them because I think the knowledge may vary there.
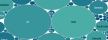 |
Read why choosing InetSoft's cloud-flexible BI provides advantages over other BI options. |
Case Study: How JobMatch, a Job Placement Services Firm, Benefited from a Business Intelligence Application
JobMatch is a mid-sized job placement services firm operating in the northeastern United States. Founded in 2005, JobMatch has built a reputation for effectively matching job seekers with employers, boasting a high success rate in placements. The firm serves various industries, including IT, healthcare, finance, and engineering, providing a crucial link between job seekers and companies looking for talent.
The Challenge
Despite its success, JobMatch faced several challenges that hindered its growth and operational efficiency:
- Data Overload: With thousands of job seekers and employers in their database, JobMatch struggled to manage and analyze the vast amounts of data effectively.
- Inefficient Matching Process: The matching process relied heavily on manual work and basic software tools, leading to inefficiencies and delayed placements.
- Lack of Insight: JobMatch lacked deep insights into market trends, candidate success rates, and client satisfaction, making it difficult to refine their services and strategies.
- Competitive Pressure: The rise of advanced job placement platforms and automated tools posed a significant threat to JobMatch's market position.
The Solution: Implementing a Business Intelligence (BI) Application
To address these challenges, JobMatch decided to implement a comprehensive Business Intelligence (BI) application. After evaluating several options, they chose InetSoft's BI platform due to its robust data mashup and transformation capabilities, which are essential for integrating and analyzing diverse data sources.
Implementation
The implementation process was carried out in phases:
-
Data Integration: InetSoft's data mashup tool was used to integrate data from multiple sources, including their existing CRM system, job boards, social media platforms, and internal databases. This created a unified data repository that was easier to manage and analyze.
-
Data Transformation: The BI application allowed JobMatch to transform raw data into meaningful insights. For example, they could segment job seekers by skill sets, experience levels, and geographic locations, and employers by industry, company size, and hiring trends.
-
Dashboard Creation: Interactive dashboards were developed to provide real-time insights into key metrics such as placement success rates, average time to placement, candidate satisfaction scores, and market demand for various skills.
-
Advanced Analytics: The BI platform's advanced analytics capabilities enabled JobMatch to perform predictive analytics, helping them anticipate job market trends and identify the best matches between job seekers and employers.
-
Training and Adoption: Comprehensive training sessions were conducted to ensure that JobMatch's staff could effectively use the new BI tools. Adoption was monitored and supported to maximize the benefits of the new system.
Results
The implementation of InetSoft's BI application brought significant benefits to JobMatch:
-
Enhanced Efficiency: The automated data integration and analysis reduced the time required for the matching process, enabling quicker placements. The firm reported a 30% reduction in the average time to placement within six months of implementation.
-
Improved Matching Accuracy: With advanced analytics, JobMatch achieved higher matching accuracy. The success rate of placements increased by 25%, leading to higher client and candidate satisfaction.
-
Deeper Insights: The BI dashboards provided deep insights into market trends, candidate performance, and client needs. This allowed JobMatch to refine their services, tailor their approaches to different industries, and improve overall service quality.
-
Competitive Edge: Leveraging BI technology helped JobMatch stay competitive in a rapidly evolving market. They could offer data-driven insights to their clients, differentiating themselves from competitors who relied on traditional methods.
-
Revenue Growth: The improvements in efficiency, accuracy, and client satisfaction translated into financial growth. JobMatch saw a 20% increase in revenue in the first year post-implementation.