InetSoft Webinar: Before You Start a Machine Learning Project
Below is the continuation of the transcript of a Webinar hosted by InetSoft in December 2018 on the topic of What Machine Learning Means for Company Analytics. The presenter is Abhishek Gupta, Chief Data Scientist at InetSoft.
I think it's just really important to think about before you start a machine learning project or an analytics project, how are you going to tell if this is making sense, if you're saving money, if you're creating revenue, if you're finding knowledge? Before you get involved with one of these projects you need to think about how you're going to assess it.
That varies a lot by different businesses but being able to have a feedback loop where you can tell how well your machine learning project did, you need to think about that from the beginning. How am I going to work that into my machine learning solution? What are my assessment criteria going to be? Am I trying to create revenue? Am I trying to find savings? Am I trying to generate knowledge? Just be aware of that, it's a hugely important part of the process, but we're running out of time and we're just going to go to questions.
All right so we have time for one to two questions. Let's lead off this one: do you have any practical examples in the area of manufacturing?
Yes, but unfortunately I can't talk that much in detail about it for confidentiality reasons since this is a real customer use case. We work with a large manufacturer of high tech devices that are used in computers and cell phones. It's an older company. They have their manufacturing process nailed down just perfectly, but they want to keep pushing that. They want to keep improving them.
#1 Ranking: Read how InetSoft was rated #1 for user adoption in G2's user survey-based index |
|
Read More |
They are using very sophisticated machine learning techniques to try to find defects or problems in their manufacturing process that wouldn't be visible through more traditional sort of manufacturing quality assessment methods.
And then the closing question: how will the job description of data scientist evolve as these tools get more sophisticated and also simpler to use?
I think that question could go a couple of different ways. There could be a wider variety of people who start getting involved in what is typically the domain of data scientists today. I think we have to be sensible and think about what kind of training did those people need to get up to speed quickly?
I think the demand for data scientists is going to grow with the amount of data that's streaming in and we have to fill that gap with bodies. How can we sensibly get those people up to speed? One way is making it more accessible in the beginning. Does this mean that all data scientists still need to be able to code? I'm a little bit old school on that, I think coding is still a very essential part of more advanced data science.
I don't dare to make a prognosis on that because I think technology has shown if anything it can go beyond our expectations. I think we're going to have to think broader about how we train the next generation of data scientists. Is the next generation who's grown up with a tablet in their hand since they were little, are they still going to be willing to code or find it interesting to code? I don't know.
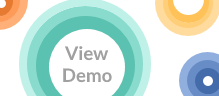 |
View a 2-minute demonstration of InetSoft's easy, agile, and robust BI software. |
Should we be teaching coding in high schools? I don't know. It's a very good question to end the debate with. I do think we're going to have to think about new ways of training the next generation of data scientist. Then you have to be creative in doing that. Only time is going to tell how important it is for a data science to be a real machine learning expert, or how important is it for a data scientist to know more about business.
Only time is going to tell what's more important. I think we'll see continued specialization of data science and data visualization, machine learning experts, data engineers, that's my prediction. But as we know, making predictions is a dangerous game in the technology industry.
We'd like to thank all of you who attended our webcast today. We hope you benefited from the presentation. Folks this will conclude our webcast today, goodbye everyone.